Couples' Behavior Multimodal Signal Processing
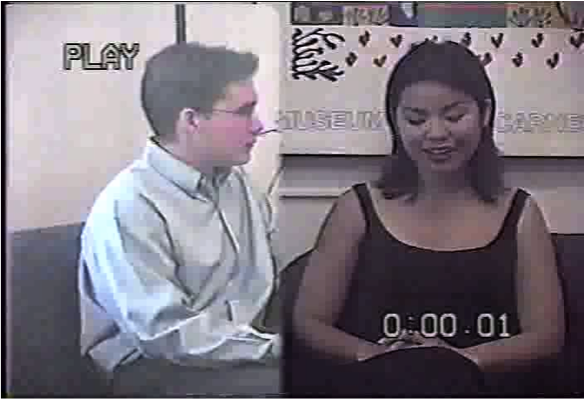
Behavioral observation is a common practice for researchers and practitioners in psychology, such as in the study of marital and family interactions. The research and therapeutic paradigm in this domain often involves the collection and analysis of audiovisual observations from the subject(s) in focus (e.g., couples or families).
In our recent work, we argue that the application of appropriate signal processing and machine learning techniques has the potential to both reduce the cost and increase the consistency of this coding process. We automatically analyze interactions of married couples and extract audio, video and transcription based behavioral cues. These low- and intermediate-level descriptors are then shown to be predictive of high-level behaviors as coded by trained evaluators. More…